ABOVE PHOTO: Assistant Professor Anne Schmitz works on computer models of mesostructures, which she is researching using AI to potentially help create better and safer consumer products.
Could artificial intelligence help ensure that fruit reaching grocery store shelves is fresh? Or make structurally stronger bike helmets? Or tires that don’t need air?
Those are a few of the possible innovations resulting from AI research by engineering professors Yuan Xing and Anne Schmitz from University of Wisconsin-Stout, thanks in part to a statewide grant and a university professorship.
Their efforts are among many AI initiatives at UW-Stout, Wisconsin’s Polytechnic University, that address the latest wave of new technology. Professors are using and teaching about AI in classrooms and labs across disciplines; Professor David Ding is serving on the Governor’s Task Force on AI; and an AI course for educators and foundational course on generative AI prompt writing are being offered.
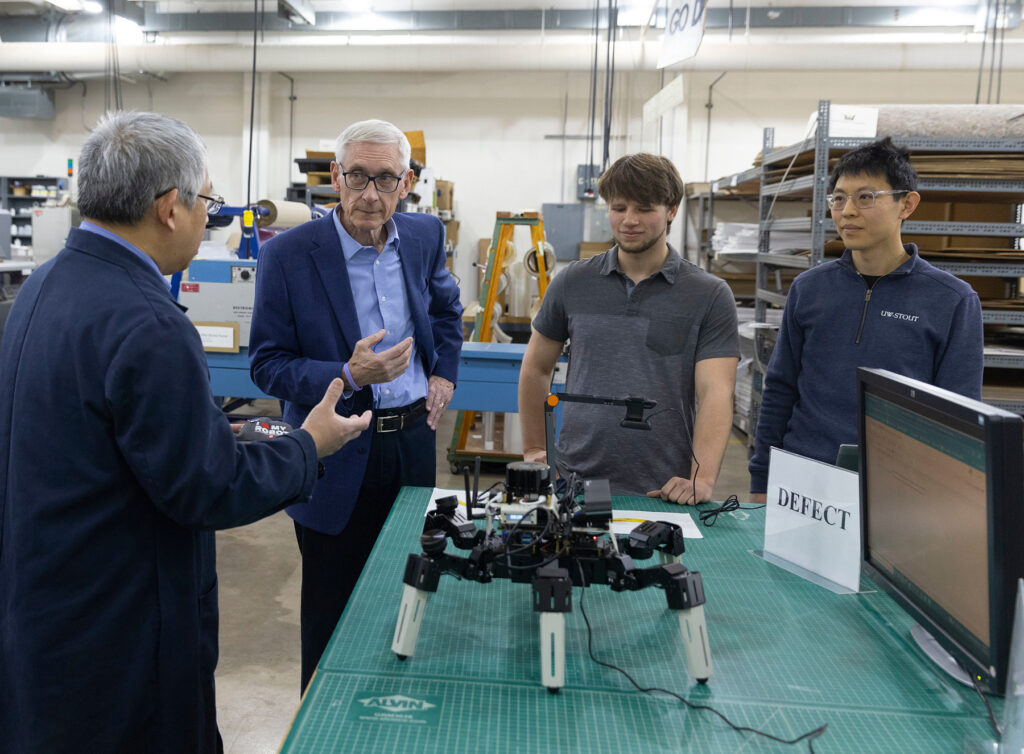
Xing is studying AI related to packaging of fruit. With his system, an automated guided vehicle in a warehouse would bring fruit to a vision sensor using a hyperspectral camera. An image of the fruit would be analyzed by an AI algorithm to determine freshness, including presence of bacteria. The vehicle then would separate the good fruit for packaging.
Supermarkets lose 8% to 10% or more of their fresh fruit because of spoilage, according to the U.S. Department of Agriculture.
Xing received a 2021 WiSys Spark Grant to develop the hardware for the small-scale, spider-like robotic vehicle, which he demonstrated when Gov. Tony Evers visited campus in spring 2024. WiSys is a nonprofit that facilitates research programs and develops and commercializes discoveries across the state for the Universities of Wisconsin.
He received two more grants in 2022, the Tommy Thompson Leadership and Freshwater Collaborative of Wisconsin awards, to complete the model.
Schmitz is conducting sustainable manufacturing research on mesostructures, or 3D-printed internal lattices that absorb energy and reduce material use. She created a computer model that predicted how the structures compress, allowing for design optimization without extensive testing. Her latest research uses AI to fine-tune mesostructure design for maximum energy absorption and lighter, more efficient products.
She conducted the research thanks to the 2021-24 Stout University Foundation’s Fulton and Edna Holtby Manufacturing Engineering Chair award, which provided $87,000.
Xing and Schmitz, assistant professors in the Robert F. Cervenka School of Engineering, explained their research in more detail:
What is the problem your research hopes to solve?
Xing: My research focuses on applying AI algorithms to improve the engineering systems in the area of automation, smart manufacturing, digital signal processing, and 5G communications. I developed multiple algorithms — machine learning models including deep neural networks, deep reinforcement learning, multiarm bandits and the associated hardware structures — including systems for AI wireless power transfer, AI driven computer vision and AI edge computing, which can be integrated into the other various systems.
Schmitz: My research tackles optimizing lightweight structures like lattices for compressive strength. Imagine airless tires or impact-absorbing foams in helmets. I’m developing a method to analyze these natural designs and fine-tune them for specific applications, all while minimizing environmental impact.
How is AI an integral part of your solution?
Xing: AI can improve the system performance by increasing the accuracy of the existing engineering systems, predicting the user pattern in the existing complicated engineering systems, detecting the defect in the existing engineering systems and planning the long-term optimization for the existing engineering systems. This work cannot be completed by other existing engineering methods.
Schmitz: Traditionally, this type of analysis relies on complex simulations and numerous experiments. However, machine learning like neural network modeling allows us to leverage AI for faster predictions. This allows for quicker iteration and optimization while minimizing the amount of material used for experiments.
Why are you confident that AI can solve this problem and others in industry?
Xing: AI algorithms are invented by simulating the human-brain and human-behaviors. So AI models have the properties of how humans solve the problems. AI models can be continuously trained and improved. If the training time is long and training is well-enforced, the model can definitely be better than the existing human-driven models. AI models can react very fast. As long as the training is completed, the AI model can be loaded to any laptop, microcontrollers and other edge devices.
Schmitz: My confidence stems from the fact that I built and trained the neural network model with reliable experimental data. The model’s predictions are then compared to known results to ensure accuracy and avoid nonsensical outputs.
How are you using AI in your classrooms at UW-Stout to help prepare the engineers of the future?
Xing: I already involve AI in most of the classes I teach. The students need to understand the principles and fundamentals of AI and utilize the models I give them to solve the engineering problems. For the higher level courses, independent study or capstone design, students even can develop their own models and implement the models in the hardware, like robots or other edge devices. My friends work in industry and can provide project ideas for students so they can learn the latest AI techniques and applications in industry.
(One of Xing’s students, Jesse Bosk, of Greenville, a senior in computer and electrical engineering, has done research into using AI to help detect knee injuries involving the ACL — anterior cruciate ligament.)
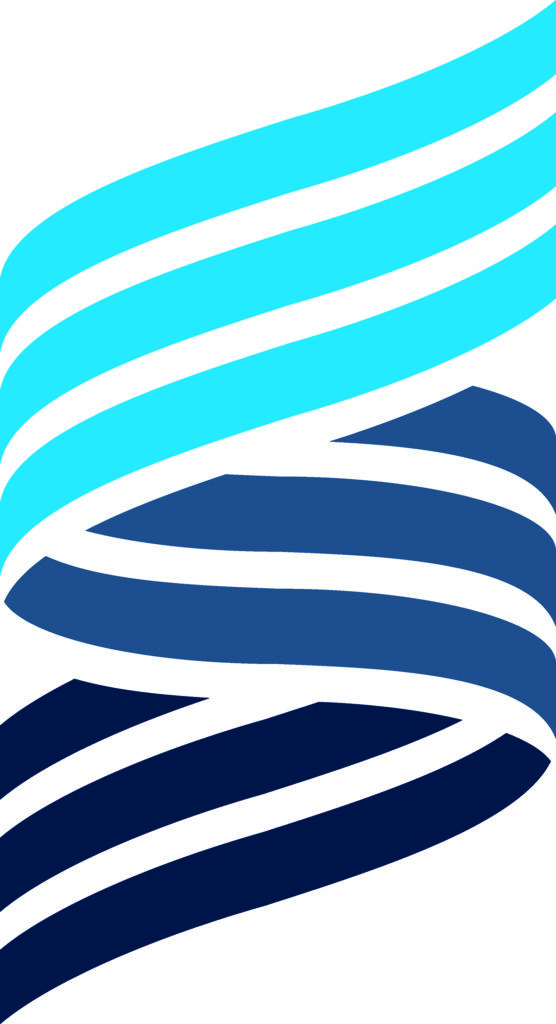